Documentos Científicos
Predictive Maintenance of Floating Offshore Wind Turbine Mooring Lines using Deep Neural Networks
We have developed a comprehensive model to help companies plan their operations in advance by detecting failure in mooring lines in almost real time using supervised deep learning techniques. Given the lack of real data, we have coupled numerical methods and OpenFAST simulations to build a dataset containing the displacements and rotations of a turbine’s floating platform across all directions.
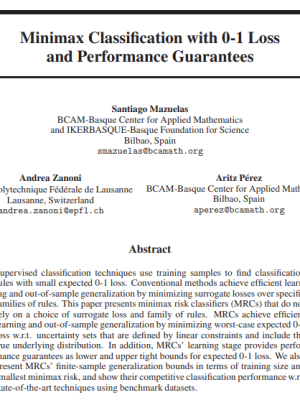
Minimax Classification with 0-1 Loss and Performance Guarantees
Los métodos convencionales consiguen un aprendizaje eficiente y una generalización fuera de la muestra minimizando las pérdidas de los sustitutos sobre familias específicas de reglas.
Este trabajo presenta clasificadores de riesgo minimax (MRCs) que no dependen de una elección de pérdida sustituta y familia de reglas.
Generalized Maximum Entropy for Supervised Classification
Este trabajo establece un marco para la clasificación supervisada basado en el principio de máxima entropía generalizada que conduce a clasificadores de riesgo mínimo (MRC).
Probabilistic Load Forecasting Based on Adaptive Online Learning
Este artículo presenta un método de previsión probabilística de la carga basado en el aprendizaje adaptativo en línea de modelos de Markov ocultos. Proponemos técnicas de aprendizaje y previsión con garantías teóricas, y evaluamos experimentalmente su rendimiento en múltiples escenarios.
La predicción de energías renovables: oportunidades Big Data para la energía eólica
El objetivo de este trabajo es mostrar la importancia que tiene realizar una adecuada predicción de la energía eólica que se va a producir, para permitir una correcta gestión, planificación y mantenimiento de los parques y del propio sistema eléctrico.
De consumidor a prosumidor
El modelo de negocio de la electricidad se enfrenta actualmente a un cambio radical,
que implica el proceso de desarrollo e implementación de las redes de energía del
futuro, las denominadas redes inteligentes o Smart Grid.
An Intelligent Procedure for the Methodology of Energy Consumption in Industrial Environments
El trabajo que se presenta en este manuscrito contribuye a la definición de los mencionados enfoques inteligentes basados en datos, definiendo un procedimiento sistemático e inteligente para el diagnóstico y la mejora de la eficiencia energética de las plantas industriales.
Big Data Architecture for Building Energy Management Systems
The enormous quantity of data handled by building management systems are key to develop more efficient energy operational systems. However, the inability of current systems to take benefit from the generated data may waste good opportunities of improving building performance. Big Data appears as a suitable framework to sustain the management system and conduct future prospective analysis. In this article, we present a Big Data-based architecture for the efficient management of buildings.
Automating look-ahead schedule generation for construction using linked-data and reinforcement learning
Look-ahead planning is the stage in construction planning where information from diverse sources is integrated and plans developed for the next six/eight weeks. Poor planning of construction site activities at this stage often results in cost overruns and schedule delays.
CntrlDA: A building energy management control system with real-time adjustments. Application to indoor temperature
Rule-Based Control (RBC) and Model Predictive Control (MPC) have been traditionally used to control building heating, ventilation and air conditioning (HVAC) systems. They, however, present shortcomings when faced with efficiently controlling these systems at a larger level. Reinforcement Learning (RL) has recently emerged as a viable alternative, showing promising results compared to previous methods, but still having some difficulties with untrained situations or sudden changes.
Representation model and learning algorithm for uncertain and imprecise multivariate behaviors, based on correlated trends
The computational representation and classification of behaviors is a task of growing interest in the field of Behavior Informatics, being series of data a common way of describing those behaviors. However, as these data are often imperfect, new representation models are required in order to effectively handle imperfection in this context.
Sinergym: a building simulation and control framework for training reinforcement learning agents
We introduce Sinergym, an open-source building simulation and control framework for training reinforcement learning agents. The proposed framework is compatible with EnergyPlus models and allows to implement Python-based controllers, facilitating reproducibility of experiments and generalization to multiple scenarios. A comparison between Sinergym and other existing libraries for building control is included.
Variational Gaussian process for optimal sensor placement
Sensor placement is an optimisation problem that has recently gained great relevance. In order to achieve accurate online updates of a predictive model, sensors are used to provide observations. When sensor location is optimally selected, the predictive model can greatly reduce its internal errors.
Minimalistic fuzzy ontology reasoning: An application to Building Information Modeling
This paper presents a minimalistic reasoning algorithm to solve imprecise instance retrieval in fuzzy ontologies with application to querying Building Information Models (BIMs)—a knowledge representation formalism used in the construction industry.
A case study on understanding energy consumption through prediction and visualization (VIMOEN)
Energy efficiency has emerged as an overarching concern due to the high pollution and cost associated with operating heating, ventilation and air-conditioning systems in buildings, which are an essential part of our day to day life.
Weak Constraint Gaussian Processes for optimal sensor placement
We present a Weak Constraint Gaussian Process (WCGP) model to integrate noisy inputs into the classical Gaussian Process (GP) predictive distribution. This model follows a Data Assimilation approach.
A time-series clustering methodology for knowledge extraction in energy consumption data
In the Energy Efficiency field, the incorporation of intelligent systems in cities and buildings is motivated by the energy savings and pollution reduction that can be attained. To achieve this goal, energy modelling and a better understanding of how energy is consumed are fundamental factors.
Attention-based Convolutional Autoencoders for 3D-Variational Data Assimilation
We propose a new ‘Bi-Reduced Space’ approach to solving 3D Variational Data Assimilation using Convolutional Autoencoders. We prove that our approach has the same solution as previous methods but has significantly lower computational complexity; in other words, we reduce the computational cost without affecting the data assimilation accuracy.
A Probabilistic Algorithm for Predictive Control With Full-Complexity Models in Non-Residential Buildings
Despite the increasing capabilities of information technologies for data acquisition and processing, building energy management systems still require manual configuration and supervision to achieve optimal performance.
Time Series Analysis for Predicting Hydroelectric Power Production: The Ecuador Case
Electrical generation in Ecuador mainly comes from hydroelectric and thermo-fossil sources, with the former amounting to almost half of the national production. Even though hydroelectric power sources are highly stable, there is a threat of droughts and floods affecting Ecuadorian water reservoirs and producing electrical faults, as highlighted by the 2009 Ecuador electricity crisis.
Generalised Regression Hypothesis Induction for Energy Consumption Forecasting
This work addresses the problem of energy consumption time series forecasting. In our approach, a set of time series containing energy consumption data is used to train a single, parameterised prediction model that can be used to predict future values for all the input time series.
Hybrid Data Assimilation: An Ensemble-Variational Approach
Data Assimilation (DA) is a technique used to quantify and manage uncertainty in numerical models by incorporating observations into the model. Variational Data Assimilation (VarDA) accomplishes this by minimising a cost function which weighs the errors in both the numerical results and the observations.
Comfort as a Service: A New Paradigm for Residential Environmental Quality Control
This paper introduces the concept of Comfort as a Service (CaaS), a new energy supply paradigm for providing comfort to residential customers.
Data science for building energy management: A review
The energy consumption of residential and commercial buildings has risen steadily in recent years, an increase largely due to their HVAC systems. Expected energy loads, transportation, and storage as well as user behavior influence the quantity and quality of the energy consumed daily in buildings.
Learning User Activities from Energy Demand Profiles
In this paper, we propose the use of energy load profiles to learn human activities. An energy load profile determines the energy consumption of an appliance during a specific interval of time. We propose the use of clustering techniques to group the different profiles according to their temporal consumption. Both Hard and Soft clustering techniques are evaluated. We have tested the method with data from REMODECE (Residential Monitoring to Decrease Energy Use and Carbon Emissions in Europe) 1 database.
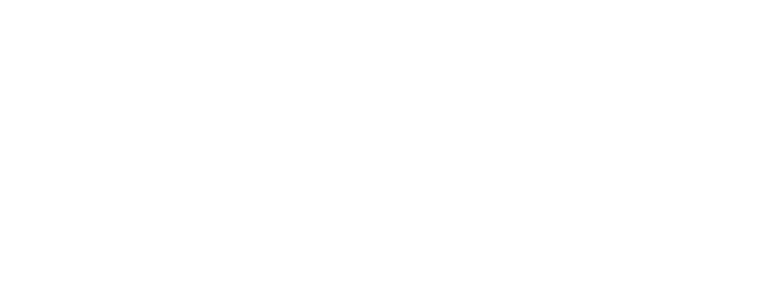
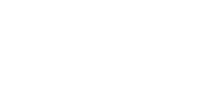
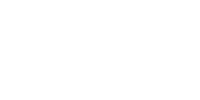
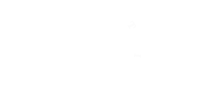